All published articles of this journal are available on ScienceDirect.
A Review of Prevalent Methods for Automatic Skin Lesion Diagnosis
Abstract
Background:
Skin cancer has been reported to be one of the most predominant forms of cancer diseases, especially amongst Caucasian descendant and light-skinned people. In particular, the melanocytic skin lesion has been judged to be the most deadly amongst three prevalent skin cancer diseases and the second most common form amongst young adults ranging from 15-29 years of age. These concerns have propelled the need to provide automated systems for medical diagnosis of skin cancer diseases within a strict time window towards reducing the unnecessary biopsy, increasing the speed of diagnosis and providing reproducibility of diagnostic results.
Objective:
This paper is aimed at using a comparative analysis method to review and compare the existing novel approaches for automating the diagnostic procedures of melanocytic skin lesion, including their success and shortcomings. This task is particularly valuable for decision makers to consider tradeoffs inaccuracy of diagnostic procedure versus complexity.
Methods:
A comparative study was carried out on selected literature from different accessible digital libraries of skin lesion research, especially cancerous moles in regard to the convention used, assumptions made, success recorded and noticeable gaps that need to be adequately filled by further study.
Conclusion:
Image standardization should be embraced in the medical research community to ensure the reproducibility of findings. Moreover, efforts should be made to have a large image library of varying skin lesion samples with categories based on lesion types and making these accessible to researchers to ensure proper benchmarking of research results.
1. INTRODUCTION
Skin cancer has been reported to be one of the most predominant forms of cancer disease, especially amongst the Caucasian descendant and light-skinned people. In particular, the melanoma has been judged to be the most deadly form of skin cancer among the three prevalent skin cancer diseases and equally adjudicated as the fifth most common cancer occurring among males, seventh most commonly occurring form of cancer diseases in females and second most common form of cancer diseases amongst young adults ranging from 15-29 years of age. These concerns have compelled the need to provide medical diagnosis within a very strict time frame through the application of advances in telecommunications-based services. Moreover, this application has been geared towards reducing unnecessary biopsy, increasing the speed of diagnosis and providing reproducibility of diagnostic results.
This study reviews the state-of-the-art approaches for achieving an automated skin lesion image diagnosis. Section 2 provides an overview of the anatomy of the skin in relation to the focus of the paper. The analysis of medical imaging in fostering a good decision-making process for skin diagnosis is discussed in Section 3. The computer-aided diagnostic system for the development of automated skin lesion diagnosis process is illustrated in Section 4. Current and state-of-the-art skin lesion diagnostic methods for assisting the diagnosis of melanocytic lesions are reviewed in Section 5. Homogenous skin lesion diagnostic procedures frequently used in the research community are comprehensively discussed in Section 6. We conclude our findings with the proposed recommendation in Section 7.
2. HUMAN SKIN
The surface of human skin is a detailed landscape with complex geometry and local optical properties. The skin is the largest organ of the human body and consists of three principal layers which are the epidermis (see 2.1 Epidermis), the dermis (see 2.2 Dermis) and the subcutaneous layer (see 2.3 Subcutaneous). Skin features depend heavily on many essential variables such as body location (forehead or cheek), subject parameters (age or gender), imaging parameters (lighting or camera) and the direction from which it is viewed and illuminated. Bacterial and viral skin infections generally affect the human skin by decolourizing and distorting the pigmented skin areas which make the automation of medical image analysis difficult [1].
2.1. Epidermis
The epidermis is a layered scale-like tissue which serves as a protection against external belligerences (extreme radiation, wounds and contaminations). The epidermis consists of four types of cells, which are Keratinocytes, Melanocytes, Langerhans and Merkel cells.
2.2. Dermis
The dermis is composed of collagen and elastic fibres. The dermis has two primary sub-layers which are the Papillary dermis (thin layer) that acts as a glue to hold the epidermis, and the dermis and Reticular dermis (thick layer) that supplies energy and nutrition to the epidermis. It contains nerve endings, sweat glands, hair follicles, blood vessels and lymph vessels. In addition, it is responsible for healing and sense of touch.
3. MEDICAL IMAGING OF THE SKIN
The principal aim of image analysis is to use image processing techniques to provide a machine interpretation of an image, typically in a format that could foster effective decision-making process. Interestingly, while the merit of medical imaging is getting popular, the World Health Organization (WHO) reported in one of its findings that three quarters of the entire world population is yet to have access to medical imaging, which is an essential technique in the new age of telemedicine such as in automation of skin disease diagnosis [2]. Hitherto, medical imaging has contributed immensely towards advancing medical procedures. However, one notable challenge is that interpretation and analysis of medical imaging results are still heavily dependent on medical experts whose availability is low or non-existent for developing and underserved regions (especially rural settings).
The fundamental task of medical imaging of the human skin is the segmentation of a mole that provides essential output for the mole feature extraction and mole classification. A mole is a skin lesion that essentially results from the local proliferation of pigment cells (melanocytes). Due to its root in melanocytes, it can sometimes be referred to as melanocytic nevus (naevus). Typically, a mole can be congenital or acquired. Congenital melanocytic nevi are present at birth and sometimes referred to as a birthmark in some regions. Congenital moles are often classified based on size. Three main types of congenital moles include small-size nevi, medium-sized nevi and giant-sized (garment) nevi. Acquired melanocytic nevi generally appears at a later stage in childhood or adult life because of several reasons such as unprotected exposure to sun radiation, immune status, genetic factors and at times from unpredictable adverse event from medication [3]. Mole transformation from nevi into cutaneous melanoma has been reported in the literature to increase with age, especially the dysplastic nevi [4, 5]. A benign mole might grow to be cutaneous for 1 in every 200000 male and female under the age of 40, as well as for 1 in 33000 for males older than 20 years of age [4]. While most moles occurring in adolescents might not transform into cutaneous melanoma [4], it has been reported that precautions need to be taken for scheduled examination on suspicious moles because some malignant melanoma might masquerade clinically as benign lesions [6, 7].
A skin lesion could also be categorised as pigmented or non-pigmented, based on its colour resulting from melanin, blood or exogenous pigment. While most Pigmented Skin Lesions (PSL) are melanocytic (benign moles or malignant), some have been reported to be non-melanocytic [8, 9]. Most moles could be said to be benign (not harmful). A cancerous mole, however, is malignant (life-threatening). Some reports have argued that a number of malignant melanomas stem from the preexisting benign nevi [4, 10].
Pathologically, melanocytic nevi are often classified based on the location reference of the nevi cells in the skin. Dermal or intradermal nevi are associated with nevus cells located in the dermis. Junctional nevus refers to a flat mole affiliated with nevus cells located at the junction of the epidermis and dermis. Compound nevi have nevus cells at the epidermal-dermal junction and equally within the dermis. The usage of dermoscope in the process of dermatoscopy has introduced the classification based on pigment patterns. A starburst nevus reflects radial lines around the periphery of a skin lesion. Blue nevi refer to uniform but structure less skin lesions that are steel blue in colour. Other common nevi include spitz, reticular, globular, eclipse, dysplastic (atypical), fried egg, lentiginous and cockade nevus.
Early detection of malignant moles is one of the essential keys to prevent untimely death resulting from skin cancer diseases [11-28]. The three prevalent skin cancers, according to the literature are Basal Cell Carcinoma (BCC), Squamous Cell Carcinoma (SCC) and Melanoma. The incidence of skin cancer diseases such as BCC, SCC and Melanoma has also been seen to increase rapidly throughout the world and it is gradually becoming one of the predominant forms of cancer diseases, especially in Caucasian population countries and among fair-skinned people [29-31]. Skin cancer incidence is on the order of 10 to 12 in Europe, 18 to 20 in the United States, and 30 to 40 in Australia per 100000 subjects [32]. The Australian Institute of Health and Welfare (AIHW) and Australian Association of Cancer Registries (AACR) detailed that more people have had skin cancer disease than all other cancer diseases combined in the past three decades [33]. Robinson [34], reported that 1 in 5 Americans develops skin cancer in the course of a lifetime. It has been reported that approximately 40%-50% of Americans who live up to the age of 65 have a high risk of having either BCC or SCC at least once [35].
Melanoma is a skin cancer typically resulting from an unpredictable disorder in the melanocytic cells, thus causing improper synthesis of the melanin. While melanoma might account for the least amongst the three aforementioned skin cancer types, it has, however, been umpired to account for 75-79% of skin cancer related deaths [29, 36]. The literature records that Melanoma is the fifth most common cancer occurring amongst males, seventh most commonly occurring cancer in females, and second most common form of cancer amongst young adults ranging from 15-29 years of age [37, 38]. Melanoma, which is currently the third prevalent cancer in Australia, was reported to occur in 61.7 for every 100000 Australian men and 40.0 for every 100000 women [33]. In the same study, melanoma of the skin was judged to have accounted for 22800 Disability-Adjusted Life Years (DALYs) in Australia. DALYs depict years of healthy life lost either because of premature death or through living with illness or injury-bound disability. The study made by American Cancer Society (ACS) [36] has revealed that at least 1 person would likely die every hour as a result of melanoma. Similarly, the study [33] reported that melanoma of the skin accounts for 22800 DALYs in Australia. DALYs refer to years of healthy life that have otherwise been lost either as a result of illness or premature death. It has been projected that melanoma would have caused 10130 deaths in the year 2016 [36] and 9730 deaths are predicted for 2017 [39].
The incidence of cutaneous melanoma in Caucasian patients has been reported to increase historically in most parts of the world over the decades [40-42]. In Europe for instance, it has been reported that malignant melanoma incidence is steadily increasing by 5% year-on-year, and it is responsible for 91% of skin cancer deaths [31]. Amazingly, most incidents are reported in the literature among Caucasians, but some reports state that black Africans and Asians account for 20% of the world melanoma [43, 44]. Tuma et al. [45], however, argued that the African descendant population is rarely affected by melanoma because an average of 1.1 out of 100,000 persons per year has an incidence of melanoma. Though most reports of melanoma have majorly reflected an infection rate among Caucasians, the overall five-year melanoma survival rate for African-Americans and other people of colour is only 77% compared to 91% for Caucasians [46]. A fact sheet report compiled by Cancer Association of South Africa (CASA) [47] has stated that South Africa has the second highest incidence of skin cancer in the world after Australia.
Gruesome reports as highlighted above have led to many advances in computer-aided systems towards assisting dermatologists to administer the diagnosis of skin-related diseases. The development of automated diagnosis systems that are capable of performing some level of remote diagnosis of skin cancer diseases such as melanoma and basal cell carcinoma and equally assisting physicians in various imaging tasks have gained tremendous attention in the bioinformatics and computer vision research [48].
The efforts towards the automation of diagnostic procedures are geared mainly to improve the speed of diagnosis and to increase reproducibility of results. The automated diagnosis has helped in reducing the first-time diagnostic errors, which sometimes could be as much as 40% [49, 50].
4. COMPUTER-AIDED DIAGNOSTIC SYSTEMS
In the past decades, the literature has reported advances in computer-aided diagnostic systems that provide a more manageable solution. These propositions are geared towards the development of automated systems that are less prone to possible bias and that are often introduced in the process of diagnosis by medical experts, whose availability is low and sometimes do not exist in underserved communities [51-53]. A strong impulse has been seen in the literature to be given to the development of automated systems capable of assisting physicians in medical imaging tasks [48]. However, the presence of noise, masking structures, variability of biological shapes and tissues, and imaging system anisotropy make the automated analysis of medical images a hard task [42, 48, 51].
One of the best approaches to overcome the aforementioned challenges in automating medical imaging diagnosis is to exploit some kind of hypothetical information about the imaged structures. The information about the structures to be analysed can be anatomical knowledge about their typical appearance (such as shape and grey levels) and position or it can be statistical knowledge of their properties such as grey level of the tissues included in those structures. The images can then be classified using their morphological structure, colour, fractal and texture properties. Laws [54], transformed digital images to identify regions of interest and provided an input data set for segmentation and features detection operation. In the same study [54], operations such as thresholding, morphological analysis and texture detection were used in order to divide a digital image into individual objects to perform a separate analysis of each region.
Over the years, it has been reported that an automatic data analysis used for melanoma showed a higher diagnostic performance compared to an observation by a physician in terms of sensitivity (proportion of true positives), though lower in terms of specificity (proportion of true negatives) [29, 55-57]. (Fig. 1) highlights the frequently used evaluation metrics to determine the effectiveness of the diagnostic results. A common technique used for the foregoing automated data analysis is Dermoscopy or Epiluminiscence Light Microscopy (ELM). It is an in-vivo, non-invasive technique that in recent years has disclosed a new dimension of the clinical morphological features of Pigmented Skin Lesions (PSLs) using different light magnification systems [29]. Dermoscopy can be based on non-polarized light techniques that require liquid interface or direct skin contact or polarized light techniques [58]. For the past decades, dermoscopy has been a major tool used by the dermatologists to proffer early detection of skin cancer-related cases, thus lowering the number of excisions and consequently impacting the clinical management of PSLs [59]. Dermoscopy provides dermatologists with a higher accuracy for detecting suspicious cases than it is possible with popular practice of naked-eye inspection [56, 60]. In addition, dermoscopy has been observed to aid the diagnosis of several other skin tumours such as Angiomas, Basal Cell Carcinomas, Cylindromas, Seborrheic Keratosis, and Hematomas, just to mention a few. In relation to the malignancy classification of melanocytic images, the ELM has been a great tool for dermatologists distinguish between life-threatening (malignant) and benign melanocytic lesions. The trend identified in the literature is the increase in the adoption of dermoscopy, primarily because of its ease of use, non-invasive approach, and slow adoption of other advance diagnostic technologies by many dermatologists. A recurring challenge, however, with the usage of dermoscopy is the complexity and subjectivity that characterize the interpretation of its results [41, 42, 57, 61, 62]. The poor reproducibility of an analysis made with the usage of the technique is also a concern.
The development of automated diagnostic systems for skin lesion screening has provided promising reproducibility of diagnostic results, and an increase in the speed of diagnosis procedures [42, 63, 64]. In addition, the application of automated diagnosis has assisted to reduce the first-time diagnostic error which can be as much as 40% [49, 50] and mis-pathology cancerous analysis [65]. Proposed automated diagnosis techniques in the literature are essentially based on different diagnostic checklists and rules such as the Asymmetry, Border Irregularity, Colour (ABCD) variation and diameter of lesion [14], modified ABC-point list of dermoscopy [66], pattern analysis [67], ELM 7-Point checklists [15], and Menzies score [68].
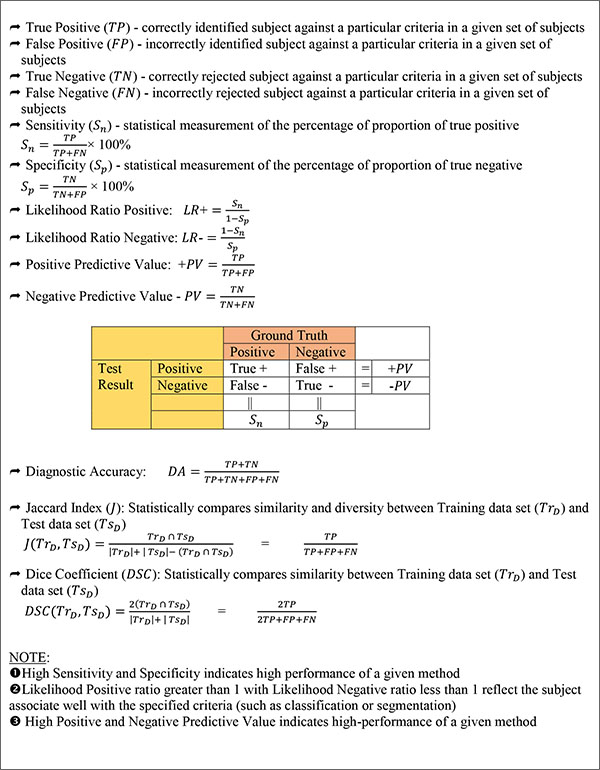
5. SKIN LESION DIAGNOSTIC METHODS
The literature generally shows that several methods for skin lesion diagnostic have been proposed to assist in the diagnosis of melanocytic lesions over the years. Prominent among these methods are Pattern Analysis for Microscopic Images (PAMI) [67], the ABCD criteria for macroscopic images [11], the ABCD rule of dermoscopy [14] for microscopic images, the ABCDE criteria [19] for macroscopic images, ABCDE rule [12], Glasgow 7-point checklists [13, 69] for macroscopic images, ELM 7-point checklists [70] for microscopic images, Menzies score [68] for microscopic images, 7 features for melanoma [71], Modified ABC-Point (MABCP) list of Dermoscopy [66] and Colour, Architecture, Symmetry, and Homogeneity (CASH) algorithm [72, 73].
The quantitative pattern analysis proposed by Pehamberger et al. [67], is based on detailed qualitative assessment of the numerous individual ELM criteria and typically requires a significant degree of formal training. Pattern analysis categorises specific patterns as global (reticular, globular, homogeneous, parallel) or local (pigmented network, dots, streaks, globules, blotches). The ABCD criteria proposed by Friedman et al. [11] employs a semi quantitative counting classification based on the evaluation of asymmetry of overall lesion shape, border irregularity, colour variation and diameter of lesion of minimum of 6mm. The ABCD rule of dermoscopy initially suggested by Stolz et al. [14] and later standardized in Argenziano et al. [52] uses similar measures in relation to the criteria defined by Friedman et al. [11], although different. Stolz et al. [14] have highlighted the key features of diagnosing a skin lesion. These features include asymmetry properties of the specific lesion (contour, colour and structures), unexpected border sharpness, colour variegation of 1 to 6 predefined colours (white, red, light brown, dark brown, blue-grey, black) and the inclusion of 5 differential dermoscopic structures (network, structure-less or homogeneous areas, branched streaks, dots, and globules). It was recommended that white colour should be only counted if the area is lighter than the adjacent skin. A Total Dermoscopic Score (TDS) of 4.75 or less signifies a benign melanocytic lesion, a score ranging from 4.8 to 5.45 denotes a suspicious lesion, and a TDS of more than 5.45 symbolizes malignancy.
Blum et al. [66] debated the need to simplify the criteria used in identifying malignant lesions. The simplified procedure termed as ABC-point (ABCP) list was formulated based on the concept of the ABCD rule of dermoscopy [14], Menzies score [68], and the modified ABCD rule by Kittler [56]. The simplicity of the ABC-point list for lesion evaluation is a great benefit, however, there exist some concerns about its sensitivity and accuracy. The CASH algorithm for dermoscopy proposed by Henning et al. [72] suggested that architectural order of lesion could be the most important features in distinguishing between malignant and benign melanocytic lesions. The comparative study carried out for CASH and state-of-the-art methods (Menzies score, ABCD rule of dermoscopy and ELM 7-point checklists) reported a comparable result [73].
Recently, a modified 4-points algorithm designed on the success of ABC-point list has been proposed, whose accuracy is similar to the CASH algorithm and similar in simplicity to the 3-point checklist [74]. The 4-point algorithm uses the existing criteria from the ABC-point list and adds another criterion by doubling the symmetry parameter criterion. The algorithm certainly looks promising, it might however be difficult to really ascertain the superiority of the algorithm over the ABC-point list and CASH, given the small sample size on which it was tested. Moreover, the validation of this new algorithm is yet to be discussed in the literature.
The Glasgow 7-point checklists which was first discussed by Mackie [13] before being popularized [69] uses change in shape, size and colour of skin lesions as its major criteria, while lesion inflammation, crusting or oozing, sensory change or Pruritus and minimum diameter of 7mm were used as minor criteria. While the Glasgow 7-point checklist has shown good adoption in clinical practice, there have been some concerns about its application in early lesion detection as well as its sensitivity and capability [15, 75]. Walter et al. [76] argued that the application of weighted revised version of the 7-points checklist, with a cut-off score of 4 rather than 3 performs considerably better and could thus be applied in general practice towards supporting recognition of clinically significant lesions as well as early identification of melanoma. ELM 7-point checklist proposed by Argenziano et al. [70] and endorsed in Malvehy et al. [61] uses 3 major criteria and 4 minor criteria, with each major criterion having a score of 2 points, whereas each minor criterion is given 1 point. A minimum total score of 3 is required for the diagnosis of melanoma. The major criteria used in the ELM 7-point checklists include atypical pigment networks, atypical vascular patterns and blue-white veil, while the minor criteria consist of irregular streaks, irregular globules or dots, irregular blotches and regression structures.
Contrary to the general adoption of the ABCD criteria for macroscopic image evaluation, there have been a number of concerns regarding the unwarranted biopsy because of misdiagnosis resulting from morphological overlap with dysplastic nevi. The relevance of the metrics such as Diameter (D) identifier from the ABCD criteria on melanoma having diameters less than 6mm or on thin melanoma (≤1 mm) has also been questioned [19, 77-82]. Whiteman et al. [83] recently validated this assumption by arguing that more melanoma deaths were attributable to thin tumours (≤1 mm) than thick tumours (>4 mm) in Queensland, Australia.
The discussions by Zaharna & Brodell [84] as well as by Liu et al. [85] reasoned that change in lesion characteristics is one of the most important diagnostic features reported by patients towards early detection of melanoma. This inference further validates the choice of variegation in size, shape, and colour as major criteria for Glasgow 7-point checklists. The literature has thus seen various proposals for additional measures to complement the ABCD criteria. Fitzpatrick et al. [12] discussed the importance of expanding the ABCD criteria to ABCDE by studying the elevation (E) of lesion for early melanoma detection. The study by Rigel & Friedman [86] and Thomas et al. [87] agreed on the need for the addition of identifier E to represent enlargement of lesion relative to other neighbouring lesion for optimizing the sensitivity and specificity of lesion diagnosis. Hazen et al. [16], equally suggested yet another similar criterion: E for evolutionary changes in lesion colour, including surrounding erythema and hyper-pigmented halo, size, pruritus, pain, surface characteristics, bleeding, symmetry and tenderness. To avoid misinterpretation of terms and to further ease distinguishing between melanoma and benign pigmented lesions, Abbasi et al. [19] proposed a more encompassing and simple criterion named evolving (E) to emphasize changes in lesion characteristics over time. Abbasi et al. [19] argued that the usage of E to represent lesion elevation (proposed by Fitzpatrick et al [12]) would be misleading since substantial elevation might not be apparent especially in early melanomas. In addition, there has been a recent discussion on the replacement of Diameter (D) in the ABCDE with lesion darkness (D) for early melanoma detection [26].
The Ugly Duckling (UD) sign introduced by Grob & Bonerandi [88] has also been seen as a major insignia for spotting the possible presence of melanoma. The UD sign signifies suspected lesions that appear different from other benign lesions examined in the same patient. The validity of the UD sign was inspected in Grob et al. [89] as a useful tool for lesion expert towards second diagnosis opinion as well as for general population when performing self-examination. The UD sign has influenced a number of research efforts towards early detection of malignant lesions. Hazen et al. [16] used the basis of UD sign to argue that it is beneficial to add another criterion of F (funny looking lesions) to the established ABCDE criteria. Similar argument to expand ABCDE to ABCDEF was recently discussed by Jensen & Elewski [90] to improve patient self-screening examination, which has been applied as a useful tool for physicians in identifying worrisome melanocytic lesions. The progressive increase in letter addition to the established ABCD criteria has been seen to have contributed to the handling of edge case skin lesion diagnosis as highlighted above. However, it has also been sometimes criticized [91].
The 7 features for melanoma developed by Dal Pozzo et al. [71] include dermoscopic features that can aid screening of pigmented skin lesions. 4 of these features are considered major, each with the score of 2, while the remaining 3 features are classified as minor features with a score of 1. The major features include regression erythema (white-pinkish depigmented area), radial streaming, grey blue veil, and irregularly distributed pseudopods. Inhomogeneity of two or more dermoscopic features, irregular pigment network and sharp margin all constitute the minor features. The 7 features for melanoma use a scoring system similar to ELM 7-point checklists however differ in the criteria.
Menzies et al. [68], discussed 11 features required to successfully diagnose a skin lesion. 2 of the features are tagged negative, while the remaining 9 are positive. The negative features include symmetry of patterns and singular colour (either of black, grey, blue, dark brown, tan and red). The positive features include blue-white veil, multiple brown dots, pseudopods, radial streaming, scar-like depigmentation, peripheral black dots/globules, multiple (5-6) colours, multiple blue or grey dots and broadened network. According to Menzies’ score, a lesion is considered melanoma if it contains 1 or more of the positive features and none of the negative features.
In a bid to effectively recognize acral melanoma that does not exhibit the parallel ridge pattern, Lallas et al. [92] recently proposed irregular Blotch, parallel Ridge pattern, Asymmetry of structures, Asymmetry of colours, parallel Furrow pattern and Fibrillar pattern (BRAAFF) as a new checklist to improve diagnostic sensitivity of the acral melanoma. The BRAAFF checklist is composed of four positive of irregular blotches, ridge pattern, asymmetry of structures and asymmetry of colours and two negative predictors of furrow pattern and fibrillar pattern.
A comparative analysis made by Annessi et al. [93] on three of the algorithmic methods (Pattern Analysis, ABCD rule and 7-Point Checklists) using 198 equivocal melanocytic lesions revealed that Pattern Analysis was the most sensitive (85.4%) and specific (79.4%) in identifying Thin Melanoma (TM), followed by ABCD rule. Comparative performance of 4 dermoscopic algorithms (pattern analysis, the 7-point checklist, the ABCD rule, and the Menzies method) by non-experts for the diagnosis of melanocytic lesions lauded Menzies method for producing the highest diagnostic accuracy [94]. Over the years, dermatologists have been using both ABCD criteria as well as the ABCD rule as a standard for classifying Pigmented Skin Lesion (PSL) as benign, suspicious or life threatening (malignant) primarily because of their simplicity and efficient approach [66, 95, 96].
It is important to note that the rules that target microscopic (dermoscopic) images differ from that of macroscopic (clinical) images even in the areas where similar terms are shared. The ABCD criteria [11] for macroscopic images differ from ABCD rule of dermoscopy [14] for microscopic images. The identifier ‘B’ in the study of Friedman et al. [11] refers to border irregularity, whereas the same identifier reflects border sharpness in the study of Stolz et al. [14]. In addition, identifier ‘D’ refers to differential structure for microscopic images, whereas it generally represents diameter greater or equal to 6mm in macroscopic images. These consequently filter down to the popular ABCDE criteria [19] and likewise the ABCDE rule [12]. Similarly, the different criteria highlighted above for both macroscopic bound Glasgow 7-point checklists discussed by Mackie & Doherty [69] and the microscopic bound ELM 7-Points checklists proposed by Malvehy et al. [61] showed a clear distinction between criteria and checklists used in both the procedures.
Most articles in the literature generally use either of aforementioned methods in speculating lesion classifications. This speculation is often accompanied with the assumptions that malignant moles are pigmented. However, there has been an increase in the reports of non-pigmented skin tumours [97-100]. This suggests a more careful approach and systems that need to be instituted to resolve such cases to curtail potential fatality. It should also be noted that some types of melanoma (amelanotic) have been reported to be clinically and dermoscopically featureless resulting in misdiagnosis during both clinical examination and dermoscopy screening [101].
6. HOMOGENOUS SKIN LESION DIAGNOSTIC PROCEDURES
To achieve a reproducible diagnosis, the research community has frequently used a number of standard automated procedures for improved diagnosis of Pigmented Skin Lesions (PSL) and its non-pigmented counterpart. These procedures include skin lesion image acquisition and preprocessing; lesion segmentation from surrounding healthy skin, extraction of selected features and classification of skin lesions.
6.1. Skin Lesion Image Acquisition and Preprocessing
Results of diagnosis reported in the literature have been judged to be highly dependent on the volume and quality of images used [29, 102, 103]. Often, variations in devices used in capturing lesion images and conditions under which these images are acquired have been observed to adversely affect the results of automated skin lesion diagnosis. In the time past, the source of image data for lesion screening was colour slides. However, over the past decades, it has been proven that quality and accurate diagnosis can be achieved using digitized lesion images [104-106]. The two predominant dermatological image types are macroscopic (clinical) and microscopic (dermoscopic) images. While the use of digitised dermoscopic images is on the increase, some reports have argued that pertinent distinguishing image features (diminishing textures and pored) are easily examined using macroscopic images rather than under dermoscopic images [107].
The literature has reported several imaging techniques that could assist in the acquisition and screening of skin lesion images [108-110]. One of such popular technique is dermoscopy which provides in-vivo, non-invasive imaging of skin lesion using different light magnification systems [14, 17, 45, 52, 56, 58-61, 66, 67, 111-131]. Other notable imaging techniques include digital photography [108, 110, 131-134], radiography [110, 135], confocal microscopy [80, 108, 115, 133, 136-148], tomography such as computed tomography, positron emission tomography, photoacoustic tomography, optical coherence tomography and magnetic resonance imaging [108, 110, 149-172], ultrasound imaging [108, 110, 173-180], multispectral imaging [108, 181, 182] and thermal imaging (thermography) [183-186]. A review of non-invasive imaging techniques was recently discussed by Menge & Pellacani [109], detailing the application of various imaging techniques and the accompanying shortfalls. Arguably, due to slow adoption of advances in diagnostic technology by many dermatologists, the trend noticed in the literature is a growing increase of the usage of dermatoscopy (Dermoscopy). Recently, the usage of dermatoscope with mobile phone camera has also been discussed in some studies for making acquisition of lesion images easier [28, 187, 188]. Reflectance microscopy has equally been dubbed to give good result against the light coloured melanoma lesions [80].
While each individual imaging method has produced a promising result in the screening of lesions, there has been a rise in the mixture of imaging methods to enhance sensitivity, specificity and accuracy of lesion screening [151, 152, 189, 190]. This is further validated by Mohr et al. [191] and Reinhardt et al. [153] and recently by Bourgeois et al. [170] that the combination of Positron Emission Tomography and Computed Tomography (PET/CT) revealed a better sensitivity in staging of malignant tumours. Wang et al. [173] equally argued that integrating photoacoustic tomography with ultrasound has yielded a better specificity when compared to when either method was used in isolation. The combination of confocal and photo thermal microscopy was recently discussed by He et al. [192] for noninvasive and label free 3-D imaging of melanoma. A good review was conducted by Dancey et al. [110] to compare various techniques used in imaging melanoma, and consequently recommended a choice of imaging techniques based on their applicability, accuracy and cost. In the review [110], it has been suggested that ultrasound imaging (ultrasonography) is the most effective mode of screening in the absence of sentinel lymph node biopsy. A similar view was shared by Xing et al. [189] during the comparison made between the usage of ultrasonography, CT, PET and PET/CT in staging and surveillance of melanoma patients.
In image processing, commonly used colour spaces include Red-Green-Blue (RGB and sRGB), Commission Internationale de l'Eclairage (CIE L*a*b, CIE L*u*v and CIE X*Y*Z), Luma plus chrominance (Y’CbCr, Y’PbPr, Y’UV and YIQ) and Hue-Saturation-Intensity-Value-Luminance (HSI, HSV/B and HSL). Most digitized lesion images are commonly generated as RGB. However, because of device dependency of RGB colour space, digitized lesion images are often converted to greyscale or blue channel for single channel (scalar) processing in order to represent the intensity of the image. In a bid to ease the accuracy of classification, Dobrescu et al. [48] converted each image used in their study to 256 grey levels image of the same size as a form of preprocessing of the image in Hue Saturation Value (HSV) colour space. Multichannel (vector) processing can equally be used to take advantage of the original colour information of the lesion. The main challenge, however, with the use of vector images is the computational requirement. Gómez et al. [193] argued that it is implausible that a particular colour space is optimal across different dermoscopic images acquired via different systems, even though the images have similar prognosis. Some reports [194-196], however, revealed that CIE L*a*b colour space produced a convincing result compared to its counterparts (CIE L*u*v and CIE X*Y*Z) and the popular YCbCr colour space when performing preprocessing of multichannel microscopic lesion images.
The term preprocessing in lesion image diagnostic procedures usually encompasses lesion image enhancement, image restoration with neighbourhood pixels and artefact removal [197]. The conditions surrounding the acquisition of lesion images generally influence possible discriminating features that can be extracted from such images for the purpose of automated diagnosis. Rahman et al. [198] reasoned that retrieval and the classification tasks of lesion could be challenging when images collected from separate data sets are captured by different devices under varying conditions (such as lightening). This creates a non-uniform illumination pattern, thus confusing diagnostic procedures. Colour calibration of image acquisition device has been one of the approaches proposed in the literature to resolve such challenges [199-205]. Low contrast of lesion images could also make isolation of lesion a very difficult task [206]. Abbas et al. [195] proposed enhancing lesion image contrast by adjusting and mapping the intensity values of the lesion pixels in the specified range in CIE L*a*b colour space. One major flaw of contrast enhancement is over amplification of noise in the region having relatively small intensity range. The use of Contrast Limited Adaptive Histogram Equalization (CLAHE) might be applied to address such limitations [207, 208]. (Figs. 2 and 3) respectively illustrate the normal and filled histogram of the image shown in (Fig. 4 and 5) shows an equalized histogram of the same image in (Fig. 4) for better noise removal resolution.
A major hindrance to a successful diagnosis in medical skin imaging is the presence of artefacts, typically referred to as noise. Artefacts such as hair shaft (Figs. 6 and 7), dermoscopic gels, thin blood vessel, shadows, ruler marking, specular reflections, vignetting and air bubble can confuse diagnosis and impede achievement of better accuracy in automated diagnosis process [107, 209-211]. To resolve the challenges posed by these artefacts, the literature report the use of a number of approaches which consist primarily of artefact detection (Fig. 5) and subsequent artefact removal (Fig. 4). Methods used for aiding the detection of artefact include filtering (curvilinear matched, Prewitt, Gaussian, median and bilateral), derivative of Gaussian, morphology operations (closed based top hat) and anisotropic diffusion. Filtering is a popular method to smooth a lesion image before detecting artefacts. Bilateral filtering has been seen to perform very well amongst other types of filtering because of its edge-preserving smoothing operation on the lesion images, especially on microscopic images [194]. Karkunen-Loéve is another method often used to preserve artefact edges during image smoothening. Prominent among the artefact removal methods is the linear interpolation [212, 213]. This was popularized in the demonstration of the system named DullRazor that was proposed by Lee et al. [213] to remove hair artefacts from a given lesion image. Other commonly used artefacts removal methods include inpainting (partial differential equation, exemplar-based, fast marching) [214-219] and region growing [107, 220]. A promising method called lacunarity algorithm which is a measure of transitional invariance for computing aspects of patterns exhibiting scale-invariant changes in the structure was equally proposed by Gilmore et al. [96] to avoid the need for a more sophisticated method.
The hair shafts and ruler marking appear to be the most common artefacts reported in the literature [210, 214, 221-226]. In our study, we observed that much effort has been given to the removal of hair shaft and ruler markings from lesion images [107, 209, 210, 213, 214, 220, 225, 227]. An excellent review by Abbas et al. [55] discussed a comparative study of the state-of-the-art algorithms for automatic detection of hair and restoration, vis-à-vis their applicability to the texture-part of lesion images. A novel algorithm comprising of morphological and fast marching schemes was also suggested in a study [55]. Similar procedure of using fast marching inpainting was discussed by Okuboyejo et al. [194] towards improving the speed of preprocessing of dermoscopic lesion images. Toossi et al. [228] also suggested the usage of multi-resolution coherence transport inpainting based on wavelet-based structure for the removal of hair artefacts in dermoscopic images. The algorithm proposed in a study [228] combines simple coherence transport inpainting with a wavelet decomposition and reconstruction method in an iterative and multi-resolution structure.
6.2. Lesion Image Segmentation
The successful segmentation of skin lesion from the healthy surrounding skin is a pertinent requirement for a workable lesion diagnostic process. The analysis of a number of the dermoscopic features (asymmetry, border sharpness) and clinical features (asymmetry, border irregularity) is only as accurate as the estimated lesion boundary. The variations in human interpretation of manual lesion boundary tracing have equally influenced the automation of lesion segmentation procedure [229, 230]. According to the literature, the estimation of lesion border by dermatologists has been reported to depend upon higher-level knowledge, leading to poor reproducibility of segmentation results [231]. However, Silletti et al. [232] argued that with exception of the Fuzzy C-Means (FCM), some state-of-the-art automatic segmentation methods performed poorly when compared with segmentation carried out by expert dermatologists. In Fig. 8), an example is shown on a segmented lesion image that has been localized from its surrounding healthy skin.
The segmentation task has sometimes been referred to as one of the most difficult tasks in medical imaging. Among other concerns, the difficulty can be attributed to low-contrasts surrounding the skin, fuzzy borders, the existence of artefacts and irregular structures characterizing lesion images [48, 65, 211, 233-235]. Readers can refer to the previous section detailing preprocessing techniques for image contrast enhancement and removal of occluding artefacts typically found in both macroscopic and microscopic images. Some reports in the literature have equally suggested that tumour areas manually extracted by dermatologists have been discovered to be sometimes characterized with inconsistency [232, 236-238], validating the need for an automated lesion segmentation approach that can aid reproducibility of results. In recent times, the literature has seen a great improvement in automating lesion image segmentation from the surrounding healthy skin parts for the purpose of achieving automated diagnosis of such lesion images. However, Chang et al. [239] argued that it is impractical to perform fully automatic segmentation on all skin lesion images due to reasons such as complexities surrounding acquisition of lesion images.
Most segmentation approaches incorporate some forms of image preprocessing to reduce or eliminate image noises such as air bubbles, ruler marking, hair shafts that could confuse segmentation. An example of this is the application of combined spline and B-spline by Abbas et al. [240] to enhance the quality of dermoscopic images before segmentation. The Karkunen-Loéve Transform (KLT) also known as Principal Component Analysis/Transform (PCA/T) was used to enhance the edges of the lesion image for better segmentation result in some studies [20, 57, 193, 241, 242]. The top-hat and bottom-hat transformations were applied in a study [243] to maximize the contrast of lesion images in order to achieve a comparable lesion segmentation using ensemble methods. The literature has chronicled the numerous lesion localization (border detection) approaches that can help to segment pigmented skin lesion from the neighbouring region in an automated mode. A number of lesion segmentation algorithms (including edge based, region based and thresholding) have equally been proposed in the literature. In the course of our study, we observed that most of the reported segmentation methods in the literature are based on the colour information of the lesion being examined arguably due to the simplicity of the representation of lesion colour properties. Some reports [244-249] have equally used texture properties of skin lesions to estimate lesion boundaries. Commonly adopted texture feature methods used in segmenting skin lesion areas include Grey Level Co-occurrence Matrix (GLCM) [245], Gabor functions [248], Laws texture energy masks [54], Markov Random Field (MRF) models [246, 250]. Glaister et al. [244] equally proposed a texture oriented lesion segmentation algorithm called Texture Distinctive Lesion Segmentation (TDLS). The TDLS algorithm uses joint statistical information to characterise skin and lesion textures as representative texture distributions. Maeda et al. [251] combined colour and texture features in a proposed Fuzzy-based hierarchical algorithm to achieve a perceptual segmentation of dermoscopic lesion images.
The edge based segmentation methods essentially use metadata about edges of a given lesion image in addition to related post-processing techniques to estimate the boundary of a lesion [219, 234, 252, 253]. The implementation of edge-based lesion segmentation often requires the use of the established edge operators such as Canny [254-256], Prewitt [257], Sobel [258], Kirsch [259] and Laplacian of Gaussian (LOG) [260]. An edge-based segmentation method based on dynamic programming using CIE L*a*b* colour space was proposed by Abbas et al. [233, 234]. However, the major challenge in the application of dynamic programming is its inability to accurately detect outline of lesion in scenarios where areas belonging to the lesion are divided into multiple tumours.
Region-based methods use a seed-based approach that groups the regions according to common image properties and relative information of the neighbouring pixels [236, 261-267]. Popular region-based methods include Fuzzy-Based Split and Merge (FBSM), J-Image Segmentation (JSEG) [262, 268], Statistical Region Merging (SRM) [269, 270], Iterative Stochastic Region Merging (ISRM) [266] and watershed [226, 271]. At this juncture, we would like to state that though there exists a similarity between edge-based lesion segmentation and region-based lesion segmentation, but both are different. Essentially, region-based segmentation methods require closed boundary to properly estimate lesion borders, whereas such requirement is not essential for edge-based segmentation. It has been argued that region-based lesion segmentation sometimes leads to over-segmentation [272]. Over-segmentation can occur when the interior of a lesion exhibits multi-coloured areas. Many advances have been recorded in the literature to resolve the aforementioned challenges, thus yielding effective region-based lesion segmentation. Ma & Tavares [273] recently proposed an algorithm built on deformable model methods to define speed function on the lightness, saturation, and colour information of a given dermoscopic image in order to estimate its lesion boundary. Geometric deformable models have been posed to implicitly represent the moving curve evolution in a way that helps to obtain desirable features (such as regions and the boundaries of the skin lesions) for shape and colour analysis simultaneously [273]. Similarly, a saliency-based segmentation method was proposed by Ahn et al. [274] via measurement of sparse reconstruction errors against image backgrounds to estimate contrast discrimination between the lesion part of a given image and the surrounding healthy skin. Saliency-based segmentation techniques help to resolve the problem of target localization, such as the difficulties in segmenting lesion image with multi-coloured objects, as well as lesion images having similar colour between the foreground and background region. The approach proposed by Olugbara et al. [275] utilized a perceptual colour difference saliency with morphological analysis to achieve a compelling segmentation result of lesions. A good future research would be to investigate how saliency segmentation can be used on lesion images with multiple saliency-regions.
In general, contour segmentation methods can be either region-focused or edge-focused. Edge related contour segmentation typically applies edge detectors to estimate stopping function for terminating contours at distinct edges, making it unusable for fuzzy edges. Region related contour segmentation computes region energy based on the mean value of lesion image intensity and consequently uses global image information to terminate contours even for indistinguishable edges. Most contour-oriented lesion segmentation techniques are more or less similar to their region-based segmentation method counterparts. The similarity is due to the usage of seed-based approach in categorising image region according to the common criteria between both methods. Contour oriented segmentation is often referred to as snakes [219]. Frequently used contour-oriented methods include adaptive snake, robust snake [276], Gradient Vector Flow (GVF) snake [277, 278], Mean-Shift based GVF [279-282], level set [263, 264, 276, 283, 284] and radial search [285, 286]. Mete & Sirakov [287] discussed enhancing active contour model with optimum parameters, including high boost filtering to achieve comparable segmentation results with other state-of-the-art segmentation methods. Similarly, Ivanovici & Stoica [288] suggested that a diffusion model for colour images can be used as external energy for active contours in order to achieve lesion segmentation by independently computing diffusion at various scales. The study reported by Yuan et al. [284] equally introduced a region-fusion-based segmentation framework by combining graph partitioning methods with chan-vese level set to achieve a comparable lesion segmentation result. In addition, Kasmi et al. [289] recently proposed a geodesic active contour (GAC) based lesion segmentation method that employs an automatic contour initialization close to the actual lesion boundary. This approach was lauded to address the sticking challenge at minimum local energy spots typically caused by noise artefacts such as hair shaft. Fig. (5) displays an example of result after applying contour-based techniques called Line Segmentation Detection (LSD) [290] on an image in order to segment occludinh hair artefacts.
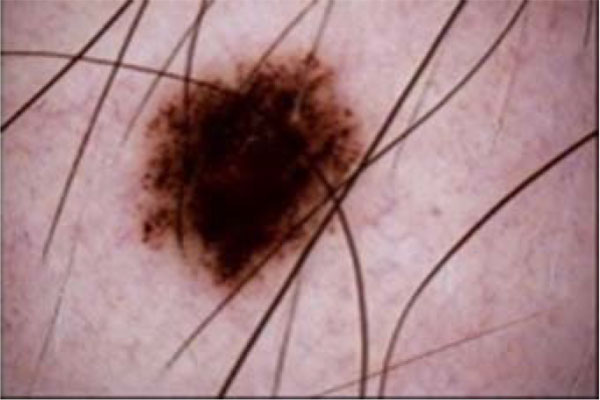
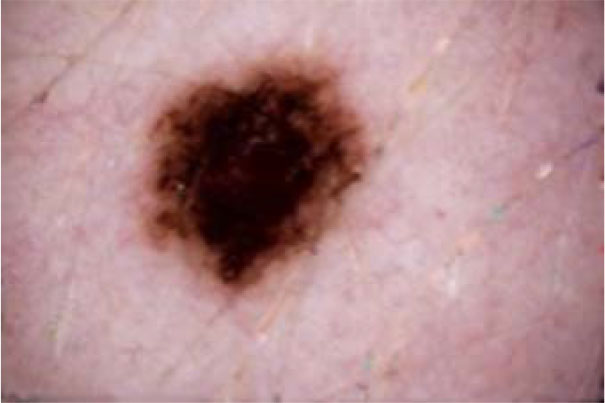
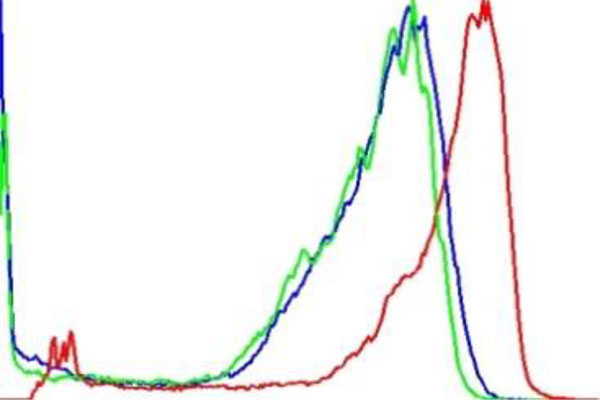
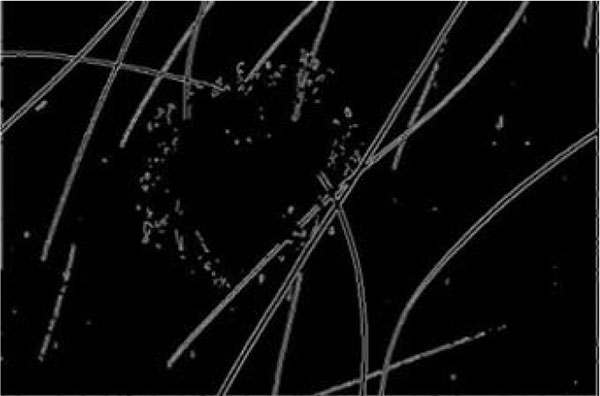
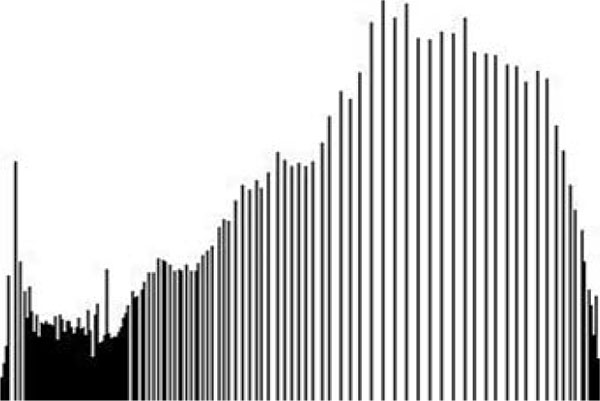
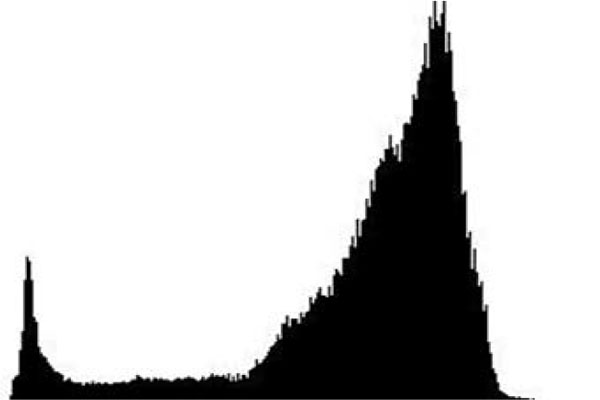
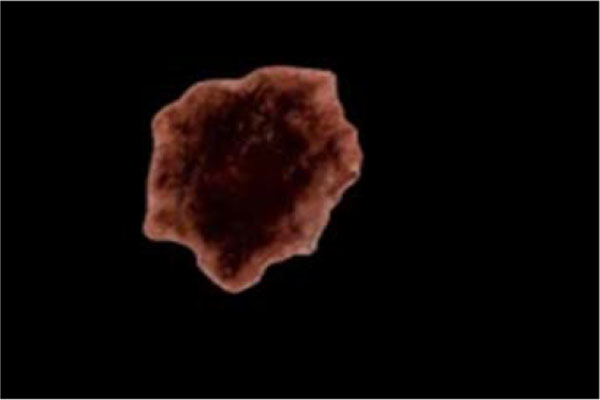
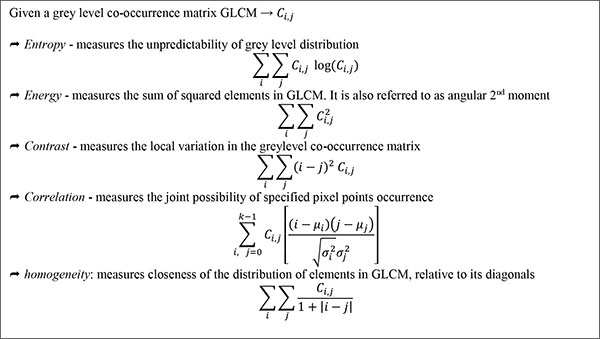
The thresholding technique is adjudged to be the most adopted approach in the literature for lesion segmentation using the computation of image intensity [198, 206, 207, 221, 240, 247, 291-299]. The discussion of thresholding in this section addresses segmentation of the lesion image rather than its usage in preprocessing of images. Typically, thresholding technique involves a non-linear process of producing a binary image such as by assigning two levels to pixels below or above a specified threshold value. Thresholding can be categorised based on the parameter usage as either parametric or non-parametric. Parametric thresholding uses a set of parameters to control fitness of the model while non-parametric thresholding estimates thresholds by optimizing objective functions such as variance-based functions (cluster variance) or entropy-based functions (cross entropy). Non-parametric thresholding can be further categorised either as global thresholding based on whether thresholding is performed on an entire lesion image using a single value or as local thresholding if a lesion image is partitioned into sub images, with each image region having their respective threshold value. Most thresholding approaches discussed in the literature are seen to be global. Global thresholding can be further classified as either a point dependent, if the threshold value is determined using grey level of each pixel of the lesion image or as a region dependent if the threshold value is determined from the local property in the neighbourhood of each pixel of the lesion image. According to the literature, the implementation of a particular thresholding technique could be based on region entropy, local lesion property, histogram shape, spatiality, image attribute similarity as well as clustering [300]. Notable thresholding techniques reported in the literature for lesion image segmentation are based on popular thresholding algorithms such as Otsu [294, 301-306], type-2 fuzzy logic [297, 307], random walker [308], Kapur [304, 305, 309], Kittler [310], Ridler [311] and Sahoo [312].
In relation to image processing, clustering is sometimes referred to as a multidimensional extension of thresholding. Clustering based lesion segmentation techniques generally adopt unsupervised erudition to identify a finite set of clusters to which image pixels would be grouped. Notable clustering methods vis-à-vis skin lesion image segmentation include fuzzy c-means (FCM) [20, 238, 313, 314], k-means [193, 215, 315-317], g-means [248], density-based spatial clustering [229, 230, 318-320], grid-based spatial clustering [318], wavelet transform [313, 321, 322] and Markov random field (MRF). Recently, Khalid et al. [321] proposed an implementation of dynamic wavelet transform based on Cohen–Daubechies–Feauveau Biorthogonal to segment lesion images. The Independent Histogram Pursuit (IHP) algorithm proposed by Gómez et al. [193] revealed the possibility of segmenting lesion images using K-means clustering technique that is agnostic of colour space of the image and the number of image bands. Kockara et al. [323] used a graph clustering segmentation technique based on the soft kinetic data structure to estimate lesion border of microscopic images and consequently segmenting the lesion images. Mete el al [230]. proposed a border-driven density-based framework to identify skin lesion border by expanding regions at borders of a cluster. This approach was further improved [229] by removing preprocessing dependency. Castillejos et al. [313] proposed an ensemble of clustering based methods to segment lesion image by exploring all colour channels. Melli et al. argued in a comparison study [324] that mean shift clustering can outperform other colour clustering algorithms (median cut, k-means and fuzzy-c means) in terms of sensitivity and specificity as the number of clusters increases. Kockara et al. [325] argued that density-based clustering produces a high precision and recall rate, with low border error when used to estimate lesion image border leading to a superior result when compared to the FCM. Recently, Lemon et al. [320] advanced the usage of density clustering by proposing a skin lesion border detection method based on web computing language (WebCL) parallel density. The approach [320] takes advantage of Graphical Processing Unit (GPU) computing power of web browsers to provide quick skin lesion border detection for dermoscopic images.
The usage of morphology and statistical information, together with clustering based approaches has equally been reported in the literature. This technique involves the use of morphological features to estimate discontinuity in lesion image structures [193, 261, 326-328]. Popular morphological based clustering methods include normalized cut [328], Principal Component Analysis (PCA) [20, 57, 193, 242], linear component analysis (LDA) [329], median cut [324] and grabcut [207].
There has been a growing need to advance lesion segmentation via machine learning system. This has led to the application of several expert systems to aid segmentation of lesion images from surrounding healthy skin [238, 322, 327, 330-332]. Application of machine learning for lesion segmentation typically involves the use of expert systems to process small areas of an image for the purpose of classification. Subsequently, the network system then classifies different areas of the image based on classifications recognized by the system. Xie et al. [331] proposed a lesion segmentation algorithm for dermoscopic images by combining Self Generating Neural Network (SGNN) with Genetic Algorithm (GA). Frequently used neural network systems recorded in the literature for lesion segmentation include Radial Basis Function (RBF), Back Propagation Network (BPN), Extreme Learning Machine (ELM), Markov Random Field (MRF) [250, 327], Wavelet Network (WN), Multi-Layer Perceptron (MLP) [326] and Bayesian [327].
The literature has equally reported attempts to use ensemble of methods to improve lesion image segmentation, such as using multiple thresholding algorithms, multiple clustering approaches, region-based segmentation with neural networks or combining thresholding with region-based methods [20, 226, 240, 243, 295, 303-305, 313, 315, 331, 333-335]. In a study [48], variable threshold (based on binary imaging) and contour extraction were used to detach the shapes of the masses before determining border outline of the lesion. A study [51] utilized Laplacian filter to localize the lesion area, while zero-crossing algorithm helped the author to perform automatic outline of the lesion border. The study [41] used both pixel-based and region-based approaches to develop an algorithm, which is referred to as Dermatologists-like Tumour Area Extraction Algorithm (DTEA) to discriminate the actual tumour area from the surrounding skin. The combination of statistical clustering of the lesion colour space and hierarchical region-growing algorithm was used in a study [336] as a segmentation technique. In another study [65], segmentation was performed using a combination of bimodal histogram based on fuzzy sets region growing. Three segmentation algorithms (global thresholding, dynamic thresholding and a 3D colour clustering concept), together with fusion strategy were used [337] to obtain binary segmentation of the lesion. Pennisi et al. [272] proposed a fully automatic lesion segmentation procedure that combined edge based method (Canny) with region-based method (Delaunay triangulation) to resolve the segmentation of lesion areas.
A number of good reviews have discussed and compared notable automated lesion segmentation approaches. In the comparison study conducted by Mete & Sirakov [287], it was argued that density-based clustering performs better than Active Contour Models (ACM) when segmenting noisy lesion images. However, the ACM was adjudged to perform better when used with an optimum parameter. Celebi et al. [338] used a normalized probabilistic rand index to evaluate five different lesion segmentation approaches which include Orientation-Sensitive Fuzzy C-means Method (OSFCM) [314, 339], Dermatologist-like Tumour Extraction Algorithm (DTEA) [40, 41], mean shift clustering method [324], modified J-image Segmentation (JSEG) method [262, 268], and Statistical Region Merging (SRM) [269]. The evaluation reported the prowess of SRM as well as the consistency of DTEA across varying lesion image types. Recently, a comprehensive lesion border detection was surveyed by Celebi et al. [340] and some of the unresolved border detection issues were discussed. The latest review by Oliveira et al. [221] on computational methods for segmenting lesion images discussed several lesion boundary techniques. In the review, edge-based segmentation for lesion image was discouraged due to the fact that edge-based segmentation doesn’t consider closed boundary, and as such may produce segmented images that are not completely closed. The comparative evaluation carried out by Mendonça et al. [276] adjudged adaptive thresholding to produce the most favorable automatic segmentation of lesions, while robust snake was said to have produced a more consistent result. Silveira et al. [283] however, argued that adaptive thresholding as well as vector-valued Chan-Vese level set [341] yielded the least satisfactory result in their comparative work. In the same study [283], a proposed extension of Chan-Vese level set, called Expectation-Maximization Level Set (EM-LS) method which uses probability density functions to model lesion intensity assumptions, was observed to produce robust skin lesion segmentation result. This inconsistency in the reported evaluation could be attributed to several factors, including varying data set used as well as different comparative evaluation metrics.
It is sometimes difficult to properly analyse different automatic border detection methods for lesion images without subjective opinions resulting from the evaluation of the parameters used. Celebi et al. [338] suggested a Normalized Probabilistic Rand Index (NPRI), which takes into account the variations in the ground-truth images when evaluating different skin lesion segmentation methods. In the study [338], NPRI was adjudged to outperform the commonly used exclusive OR (XOR) measure. Garnavi et al. [342] equally proposed a weighted performance index for objective evaluation of five automated border detection methods for dermoscopy images. The weighted index was computed from six standard evaluation metric (sensitivity, specificity, accuracy, precision, border error, and similarity). The approach was further optimized in a study [343] by applying constrained non-linear multivariable optimization method in the computation of the weights.
We observed that most reported work in the literature on lesion image segmentation has been on microscopic (dermoscopic) images. From the literature, only a few efforts have been recorded in the usage of clinical (macroscopic) images for evaluating automatic lesion area segmentation approaches [249, 261, 298, 306, 335, 344]. This arguably could be attributed to the increased adoption of dermoscope in the evaluation of skin lesion images. Cavalcanti et al. [335] proposed an Independent Component Analysis (ICA) based ensemble methods to estimate lesion areas from healthy surrounding skin. In the same study [335], ensemble of thresholding and level set methods were then applied for the actual lesion boundary detection and segmentation thereof. Recently, Flores & Scharcanski [249] proposed an unsupervised dictionary learning method called Unsupervised Information Theoretic Dictionary Learning (UITDL) for estimating lesion area in macroscopic images.
The analysis made from the reports discussed in the literature suggests that a number of past works in the lesion segmentation efforts have focused on the development of algorithms based on colour information in the non-uniform space. There’s however a growing need towards optimizing segmentation algorithms in order to reduce computation time. In a bid to resolve the later, Okuboyejo et al. [207] proposed a Fast Image Segmentation (FIS) method based on the notable Contrast-Limited Adaptive Histogram Equalization (CLAHE), morphological operations, thresholding and grabcut techniques to localize lesion area from the surrounding healthy skin in a recorded time.
While most of the segmentation techniques discussed above yielded considerable promising results, the main problem with most of the approaches is that the computer-extracted regions sometimes were often smaller than the dermatologist-drawn ones (segmentation ground truth). Consequently, this makes some areas surrounding the tumour which are important features in the diagnosis to be excluded from the subsequent analysis [96, 103]. There are indications from the literature that many existing segmentation systems have high sensitivity rates towards effective diagnosis, they however experience high computing time [41, 57]. The usage of more than one algorithm for segmentation is one of the major causes of the non-realistic computing time as highlighted in a study [57]. It has also been noted that numerous past works have focused significantly on developing algorithms based on colour information in non-uniform colour spaces (disregarding the role of textural information). This has been reported to sometimes yield unsatisfactory segmentation results [234]. Another unresolved concern is the development of clinically oriented evaluation methods that can adapt variations in multiple manual borders [340]. While future research most likely would continue to use the mixture of algorithms due to increasing success rate of such approaches, more efforts should be made towards optimizing these algorithms to reduce their computing time. We would also like to suggest that the comparison of segmentation algorithms should be done on the same set of lesion images to ensure proper accuracy measure.
6.3. Feature Extraction
The primary objective of feature extraction is to quantify the macroscopic (clinical) or microscopic (dermoscopic) signs used in determining the malignancy of a skin lesion by a set of finite numerical features. Isolation of discriminating features in a given lesion image is an essential step towards effective automated lesion image classification. However, the vast variety of dermoscopic images and highly subjective definition of features characterizing these images have made the extraction of needful features a tedious task [40, 64, 96, 345, 346]. Skin distortion caused by bacterial and viral skin infections also makes analysis of features very difficult. In addition, variables such as body location, subject parameters (age), imaging parameters (lightening or camera), and direction from which lesion image is viewed and illuminated, greatly influence the resulting features that can possibly be extracted for classification purpose. These challenges typically add some overheads towards achieving automatic screening and diagnosis of medical images, especially skin lesions.
There have been numerous attempts reported in the literature to solve some of the above-mentioned challenges. One of the best approaches to address these aforementioned challenges in automating medical imaging diagnosis is to simplify the objective of the analysis and exploit some kinds of hypothetical information about the image structures. The information about the structures to be analysed can be anatomical knowledge about their typical appearance (shape, grey levels and position) or statistical knowledge of their properties (such as the greylevel of the tissues included in those structures). The images can then be classified using their morphological properties such as colour, shape, edges and texture.
The familiarity and potential discriminating power of the previously mentioned lesion diagnostic algorithm methods (such as ABCD rule of dermoscopy, ABCD criteria and Pattern analysis) have led to their usage in feature quantification. These feature descriptors can be dermoscopic, clinical or simply morphological in nature. Most reports discussed in the literature derived various discriminating lesion feature descriptors from these diagnostic algorithms, especially from the ABCD rule for dermoscopic images and ABCD criteria for clinical images. Feature descriptors used in the discrimination of skin lesion can favourably be categorised mostly as either photometric or textural. In the literature, it has been observed as common practice to use an amalgamation of various descriptors for lesion discrimination. Essentials features with corresponding discriminating properties used across the reviewed literature has been listed in Table 1.
Photometric has been seen to constitute the majority of the properties used in the literature when examining descriptors that could be used in classifying skin lesions. Photometric features include colour, island of colour, colour homogeneity & colour histogram etc. Barata et al. [64] argued that photometric features, when used with textual descriptors, yield a good result, however, the photometric features were observed to outperform the textual features if used in isolation. Stoecker et al. [347] equally suggested in his work that greater separation of melanoma from benign lesions is achieved using relative colour than using absolute colour.
Texture-based descriptors are yet another set of features that reflect the structural pattern of lesion surfaces irrespective of the colour or illumination characterizing the lesion. Texture descriptors can be categorised as spatial frequency based, statistical based, geometric based or model based. Spatial frequency based texture features are frequently associated with wavelet and ridgelet transformations. Statistical based descriptors include co-occurrence matrices and Fourier properties for describing lesion’s local neighbourhood properties. Geometric features describe skin lesion characteristics that include shape, border, symmetry, area, diameter, variance, perimeter, circularity and anisotropy. Model based textual descriptors are frequently associated with fractals and Markov random fields. Due to simplicity and ease of feature retrieval, commonly used texture descriptors include co-occurrence texture features, wavelet features and fractal-based texture features.
Features | Properties | References |
---|---|---|
Asymmetry | asymmetry index | [27, 32, 40, 57, 62, 65, 130, 252, 253, 291, 298, 336, 344, 363, 382, 387, 388] |
circularity factor | [40, 51, 62, 267, 291, 337, 344, 349, 366, 382, 386, 388-391] | |
skewness | [103, 392] | |
Border irregularity | edge abruptness | [57, 65, 130, 252, 291, 351] |
lesion areas and perimeters | [62, 252, 253, 337, 363, 366, 367, 379, 382] | |
radial distance | [267, 369] | |
bounding box | [267, 344, 369, 377, 382, 390] | |
mean and variance of lesion boundary magnitude | [40, 57, 337, 344, 366, 393] | |
Border Sharpness | compactness index | [27, 57, 65, 252, 337, 344, 351, 379, 382, 394] |
fractal dimension | [48, 57, 65, 96] | |
Colour | colour homogeneity | [51, 57, 65, 252, 347, 351, 363] |
island of colour | [40, 51, 58, 103, 130, 366, 382, 391] | |
colour histogram | [28, 64, 198, 292, 299, 345, 366, 370, 376, 377, 379, 382, 395-398] | |
RBG statistics (such as ratio, chromaticity, spectral) | [57, 103, 252, 253, 291, 337, 344, 367, 382, 385, 392, 393] | |
Diameter | lesion diameter | [51, 57, 168, 252, 344, 369] |
Differential Structures | pigmented network (typical/atypical) | [17, 21, 28, 52, 58, 93, 94, 121, 127, 190, 242, 291, 347, 358, 371, 373, 376, 399-406] |
homogeneous areas | [61, 382, 407] | |
branched streaks globules | [17, 52, 93, 94, 345, 400, 405, 406, 408] | |
structure-less areas (such as dots, globules, blotches) | [17, 52, 93, 94, 121, 291, 345, 357, 358, 376, 400, 402, 405, 406, 408-410] | |
blue-white veil | [17, 52, 58, 121, 291, 372, 400, 403] | |
Lesion Surface Structures | co-occurrence texture features | [40, 48, 51, 103, 291, 344, 347, 351, 366, 369, 376, 382, 386, 391, 392] |
wavelet texture features | [62, 64, 336, 386] | |
Other features | correlation index between geometry and photometry | [57, 65] |
sonography characteristics, hypo-echogenicity | [57, 108, 177, 411, 412] |
Co-occurrence texture descriptors such as entropy, correlation, energy, contrast, homogeneity etc. are based on co-occurrence matrices, typically the GLCM. (Fig. 9) detailed some of the frequently used texture descriptors, as well as corresponding computation. The GLCM [348] also known as greylevel spatial dependence matrix, is a form of statistical method of examining texture in relation to image pixels. GLCM outlines within a grey scale image, the probability of greylevel ἱ occurring at a distance in direction θ from grey level ĵ.
![]() |
Wavelet texture features such as wavelet energy, variance and residual energy are based on wavelet transform coefficients. Fractal based texture features such as mean fractal dimension, local connected fractal dimension and global box-counting are based on fractal dimensions. One major shortfall of local fractal dimensions and local connected fractal dimensions, however, is the dependency on the choice of the maximum window size [48]. However, while it is desirable to determine features to represent these structures directly, extracting these features is often challenging primarily due to a vast variety of dermoscopy images and the highly subjective definitions of these features [40, 41, 96].
A number of different feature selection methods have been used in the literature towards ensuring appropriate discriminating features for lesion image classification. Frequent selection methods reported in the literature include Sequential Floating Forward Selection (SFFS) [337], Sequential Floating Backward Selection (SFBS) [337], Leave-One-Out, Cross Validation (xVal), Plus-I-Take-Away-r, and Genetic algorithm. Zagrouba and Barhoumi [57] argued that relative reduction of selection features could yield 50% reduction in the processing time, as well as 65% reduction in the time required to train classifiers.
While these selectors have produced positive results and contribute positively towards classification of lesion images, there resource intensive patterns are still a concern. There is thus a growing need to improve the algorithms implemented by each of the selectors to better achieve optimal feature selection process, which in turn would help reduce complexity and time-consuming computation experienced during quantification of features [349].
6.4. Classification of Lesion
Image classification involves using selected features of an image to classify pixels of the image into one of the several classes depending on specific knowledge domain. This could be in the form of training a model using a data set and then testing the model using a data set which is disjoint from the training set. Most lesion classifications are binary in nature, distinguishing between benign and malignant moles. The classification results are typically influenced by the chosen feature descriptors and strength of the classifiers. Performance of the automated classification is equally dependent on the degree of dataset population [168].
The two main classification types as reported in the literature in relation to medical imaging are supervised classification and unsupervised classification. Supervised classification uses image analysis tool to generate a statistical categorisation (such as mean and co-variance) of the reflectance of each identified information class. The completion of the categorisation then fosters effective classification by examining the reflectance of each pixel and deciding on the best matching signatures. Decision criterion such as maximum likelihood can be used for cases of overlapping signatures in order to assign pixels to the highest probable class. Unsupervised classification typically examines a large number of unknown pixels and divides them into a number of classes based on natural groupings present in the image values using procedures such as clustering. Essentially, unsupervised classification groups values that are close together in a measurement space as a single class, thus arranging the data in different classes to be comparatively separated [350].
The literature has reported the application of several classification methods for lesion images. Frequently used among these methods are the Artificial Neural Network (ANN), Decision Trees (DT), K-Nearest Neighbour (KNN), Support Vector Machine (SVM) and Regression Analysis (RA) classifiers. Similar to the neurons of a human brain, ANN comprises of an interconnected group of nodes, otherwise termed as neurons. Neural network models typically consist of both an adaptive weight that is adjusted during model training, as well as the capability to use quantitative characterization to approximate non-linear functions of their inputs.
Popular ANN methods include Back Propagation Network (BPN) [22, 40, 292, 332, 347, 351-355], Auto Associative Network (AAN) [22], Multi-Layer Perceptron (MLP) [57, 168, 224, 236, 349, 351, 355-357], and Single Layer Perceptron (SLP) [51, 358]. In the literature, extreme Learning Machine (XLM), SLP and MLP seem to be the most commonly used Feed Forward Network (FFN) methods. Main benefits of Bayesian network include its quick training capability and insensitivity to irrelevant features [298]. Sample application of ANN for lesion classification purpose can be seen in different studies [22, 40, 51, 57, 63, 168, 236, 292, 332, 352, 353, 359]. A major challenge in the application of ANN includes the excessive time that might be required in training dataset.
Bayesian network is another frequently used classifier in the space of lesion discrimination [351]. It is a probabilistic graphical model that applies Directed Acyclic Graph (DAG) to represent a set of random variables with their corresponding conditional dependencies. It should be noted that the term Bayesian network depicts the usage of Bayes rule for probabilistic inference and not necessarily implies commitment to Bayesian statistics. One major advantage in the use of Bayesian network is its insensitivity to irrelevant features. Its drawback, however, includes sometimes undesirable assumption declaring that discriminating features are independent [298]. Application and analysis of Bayesian network can be seen in different studies [224, 360-364]. In the literature, Hidden Naïve Bayes (HNB) has been observed to perform better than the Bayesian network method used [62, 361]. If a set of lesion outcomes represented by a vector
![]() |
Regression analysis is a statistical analysis for estimating the relationship between dependent (criterion) and independent variables (regression function or predictors). This typically tracks changes in the dependent variables as one of the members of the predictors is kept constant while other members of the predictors are varied. Frequently used regression analysis methods include Discriminant Function Analysis (e.g. Linear or Quadratic Regression) [40, 57, 60, 137, 253, 292, 364-367] and Logistic Regression [18, 75, 93, 102, 137, 368, 369].
Decision trees typically adopt a tree-like graph of possible decisions and the corresponding outcomes which could trigger another decision till a specific conclusion is reached [291, 361, 369, 370]. The major merit of using decision trees includes the speed at which it can be trained as well as its ease of use. Frequently used DT methods include C4.5 Decision Tree [291, 371-373], Logistic Model Tree (LMT) [291, 361, 374], Random Forest [357, 361, 370, 375], and Gradient Boosting (e.g. Adaptive Boosting: AdaBoost) [64, 291, 375-380]. Drawbacks seen in the usage of decision trees include difficulties in dealing with correlated features and the likelihood of over-fitting which typically results in excessive adjustments [298]. DT method was dubbed to perform the least in the comparative study described by Dreiseitl et al. [381], however, comparable to human expert.
The K-Nearest Neighbour (K-NN) is an algorithm that can also be applied as a classifier by storing the available cases and then classifying new cases based on the similar measurement in feature space [64, 198, 337, 344, 362]. The classifier input consists of k closest sample in the feature space, while its output result in class membership of objects being sampled. Contrary to some other classifiers, K-NN does not implement a decision boundary, however, uses the elements of the training set to estimate the density distribution of the data [381]. Hierarchical K-NN is an optimized subset of K-NN, however, it adopts both observation and feature space in its classification procedures.
The SVM is a non-probabilistic binary linear classifier that uses a learning module to analyse patterns within a collection of data for possible classification into one of the two categories. It adopts supervised learning for labelled data and an unsupervised clustering approach when data is not labelled. SVM also provides a unified framework in which different learning machine architectures can further be generated through an appropriate choice of kernel [382]. Applications of SVM can be seen in some studies [42, 130, 291, 354, 363, 369, 383-386]. In a number of studies, SVM was judged to outperform several classifiers [354, 357, 360, 363, 375]; and it is often praised for its good generalization and simplification of the non- linear data separation by means of kernel functions [298]. While the application of SVM in discriminating between melanocytic lesions has seen a number of good results, it sometimes could be very sensitive to noise hence producing a poor result. Contrary reports to the effectiveness of SVM when compared to other classifiers has equally been reported by some research works [64, 291]. SVM and MLP performed better than the counterpart classifier in the confusion matrix described in a study [357] between MLP, K-NN, Random Forest (RF) and SVM. In a similar study described by Dreiseitl et al. [381], logistic Regression, ANN and SVM produced good discriminating results for PSL compared to KNN and Decision Tree methods.
Kreutz et al. [336] argued for the need to incorporate a combination of expert systems in classifying lesion images to enable data set to be split into regions where each expert system works effectively. Results from each expert system can then be aggregated by a gating network. This is to help resolve recurrent challenges faced when training a single expert system to classify varying degrees of input space. In effect, when input space is separated and targeted, scalability and interpretability of solutions increase. Similarly, A Multiple Expert-Based Melanoma Recognition System for Dermoscopic Images of Pigmented Skin Lesions has been proposed by Rahman and Bhattacharya [198, 299] by using combination rules generated with the application of Bayes’ theorem to produce a probabilistic output. The comparative study discussed by Ruiz et al. [351] equally argued that collaborative classifiers produced better classification compared to the usage of individual classifiers.
Furthermore, Dreiseitl et al. [381] suggested that linear factors contribute to a better discrimination compared to non-linear elements in the classifying models. This was proved in the comparative analysis [381] between K-NN, Logistic Regression, ANN, DT and SVM, where linear method (logistic regression) outperformed non-linear counterpart. Other remarkable classifiers as reported in the literature include Lacunarity analysis [96] and Markov Random Field MRF [246]. The literature also records the use of rule-based process for classifying skin lesion images. Frequently used rule-based procedures include Pattern Analysis, ABCD rule, ELM 7-point checklists, Menzies score, 7 Features for melanoma. Notable results have been recorded in the literature by various classification methods; however, there still exists some unresolved concerns in relation to effective lesion classification. Highlights of the issues include the great unbalance between lesion image classes, the difficulty in defining discriminating visual features and the effect of multiplicities of some lesion image classes. The execution speeds of the classification algorithms and resource intensive nature of some of these classifiers have posed a need for a more optimized approach, especially when considering mobile portability of these solutions.
CONCLUSION
The development of automated systems capable of assisting physicians in medical imaging tasks has been seen to be marred by the presence of noise such as masking structures, variability of biological shapes and tissues, and imaging system anisotropy. These noises make an automated analysis of both microscopic and macroscopic images a cumbersome task. We discussed different approaches proposed in the literature for resolving some of the doubts resulting from the automated diagnosis of microscopic (dermoscopic) as well as macroscopic (clinical) images.
Most articles in the literature often assume that malignant moles are pigmented. However, there has been an increase in the reports of non-pigmented skin tumours, as well as clinically and dermoscopic featureless moles being misdiagnosed during both clinical examination and dermoscopy screening, thus necessitating a careful approach.
Among others, subjective opinions resulting from the evaluation of parameters used in lesion segmentation were recorded as one of the difficulties encountered in the literature in an attempt to analyze different automatic border detection methods for lesion images. To achieve a proper measure of accuracy and consistent results when performing lesion localization, we would like to recommend that comparison of segmentation algorithms should be done on the same set of lesion images.
We propose that more efforts should be geared towards optimizing feature selection in order to reduce complexity and time-consuming computation. A number of the classification models proposed in the literature still exhibit some challenges such as unbalance between lesion image classes, the difficulty in defining discriminating visual features and the effect of multiplicities of some lesion image classes. We believe that given a good classification model, less emphasis could be given to the number of features required to discriminate between lesion categories.
CONSENT FOR PUBLICATION
Not applicable.
CONFLICT OF INTEREST
The authors declare no conflict of interest, financial or otherwise.
ACKNOWLEDGEMENTS
Declared none.